Latent Semantic Indexing Lsi
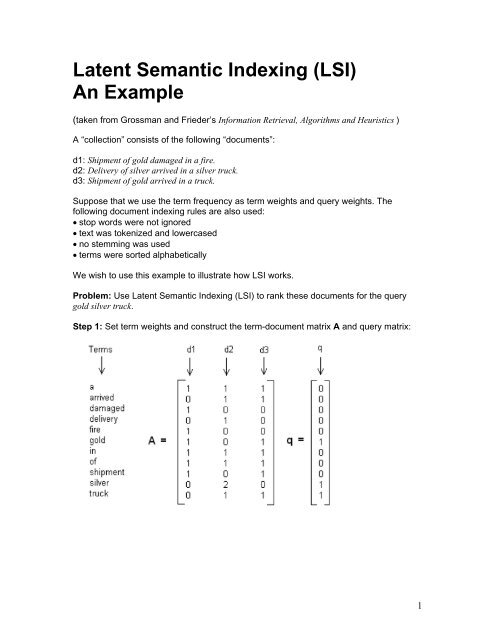
Latent Semantic Indexing Lsi An Example Latent semantic indexing (lsi) is an indexing and retrieval method that uses a mathematical technique called singular value decomposition (svd) to identify patterns in the relationships between the terms and concepts contained in an unstructured collection of text. lsi is based on the principle that words that are used in the same contexts tend. Lsa (latent semantic analysis) also known as lsi (latent semantic index) lsa uses bag of word (bow) model, which results in a term document matrix (occurrence of terms in a document). rows represent terms and columns represent documents. lsa learns latent topics by performing a matrix decomposition on the document term matrix using singular.
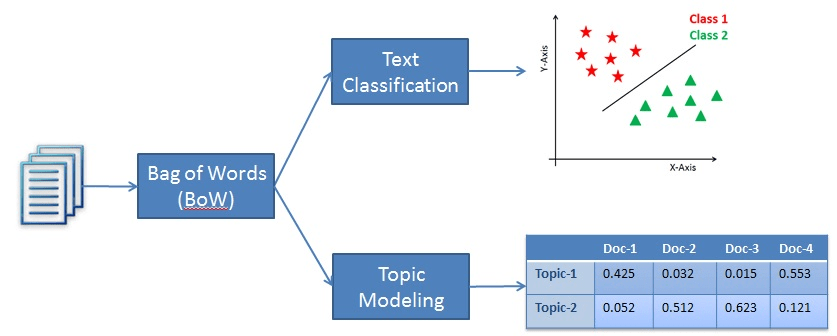
Python Lsi Lsa Latent Semantic Indexing Analysis Datacamp Lsi (latent semantic indexing) keywords are words or phrases that are conceptually related to a target keyword. so if you wanted your page to rank for “credit cards,” then lsi keywords would be “money,” “credit score,” and “interest rate.”. however, it’s more accurate to refer to these as semantic keywords. Lsi (latent semantic indexing) keywords are terms that are conceptually related to your target keywords. the technology was introduced in a 1988 paper. and is described as an “approach for dealing with the vocabulary problem in human computer interaction.”. in other words: using related words and phrases (“lsi keywords”) to better. Latent semantic indexing (lsi, for short) can be an overwhelming and confusing term for anyone hearing it for the first time. luckily, although it sounds like something that requires a degree in computer science, it's actually a concept you're already probably familiar with — particularly if you have some basic knowledge of keywords and their close relationship with search engine. The low rank approximation to yields a new representation for each document in the collection. we will cast queries into this low rank representation as well, enabling us to compute query document similarity scores in this low rank representation. this process is known as latent semantic indexing (generally abbreviated lsi).
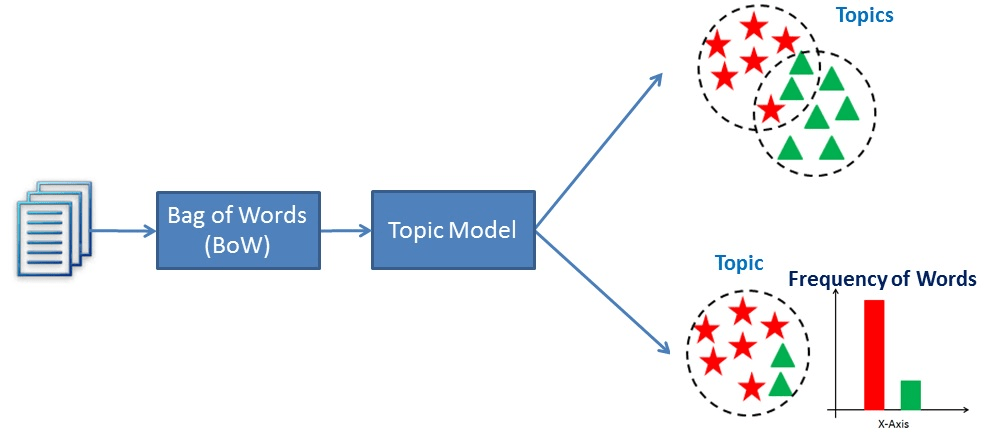
Python Lsi Lsa Latent Semantic Indexing Analysis Datacamp Latent semantic indexing (lsi, for short) can be an overwhelming and confusing term for anyone hearing it for the first time. luckily, although it sounds like something that requires a degree in computer science, it's actually a concept you're already probably familiar with — particularly if you have some basic knowledge of keywords and their close relationship with search engine. The low rank approximation to yields a new representation for each document in the collection. we will cast queries into this low rank representation as well, enabling us to compute query document similarity scores in this low rank representation. this process is known as latent semantic indexing (generally abbreviated lsi). Developed in the 1980s, lsi uses a mathematical method that makes information retrieval more accurate. this method works by identifying the hidden contextual relationships between words. it may help you to break it down like this: latent → hidden. semantic → relationships between words. indexing → information retrieval. Latent semantic indexing (lsi), or latent semantic analysis (lsa), is a natural language processing technique developed in the 1980s. unfortunately, unless you’re familiar with mathematical concepts like eigenvalues, vectors, and single value decomposition, the technology itself isn’t that easy to understand.
Comments are closed.