Latent Semantic Indexing From Scratch Lsi Presentation Pptx At Master
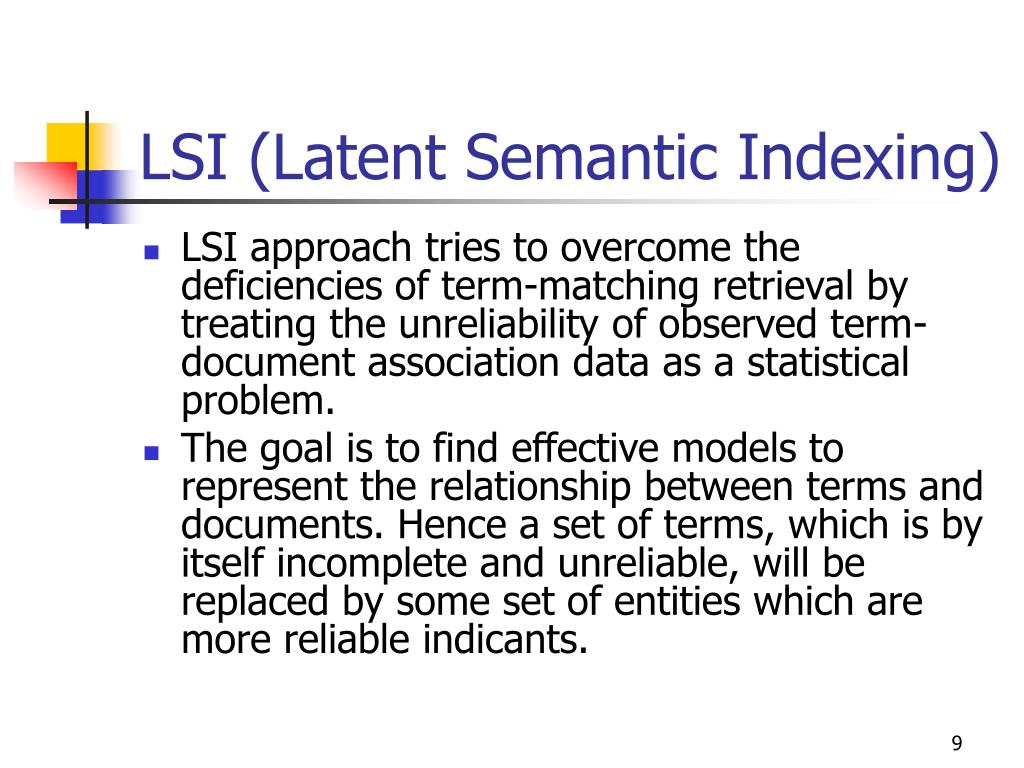
Ppt Latent Semantic Indexing Powerpoint Presentation Free Download Latent semantic indexing (lsi) or latent semantic analysis(lsa) is an indexing and information retrieval method. it is one of the major analysis approaches in the field of text mining. it helps in finding out the documents which are most relative with the specified keyword. {"payload":{"allshortcutsenabled":false,"filetree":{"":{"items":[{"name":".gitignore","path":".gitignore","contenttype":"file"},{"name":"doc1.txt","path":"doc1.txt.
Latent Semantic Indexing From Scratch Lsi Presentation Pptx At Master In this decomposition method we are looking for a set of orthonormal basis in the row space that when multiplied by the original matrix goes to an orthonormal basis in the column space.av1 = σ1u1 av2 = σ2u2 relevance ranking using latent semantic indexing from scratch lsi.pptx at master · vijeth8 relevance ranking using latent semantic. Latent semantic indexing (lsi) allows search engines to determine the topic of a page outside of directly matching search terms. lsi models the contexts in which words are used to find related pages, similar to how humans understand language through context. lsi was introduced to search engines by applied semantics and acquired by google to. Lsa (latent semantic analysis) also known as lsi (latent semantic index) lsa uses bag of word (bow) model, which results in a term document matrix (occurrence of terms in a document). rows represent terms and columns represent documents. lsa learns latent topics by performing a matrix decomposition on the document term matrix using singular. There are many different mappings from high dimensional to low dimensional spaces. latent semantic indexing chooses the mapping that is optimal in the sense that it minimizes the distance ∆ . this setup has the consequence that the dimensions of the reduced space correspond to the axes of greatest variation.1.
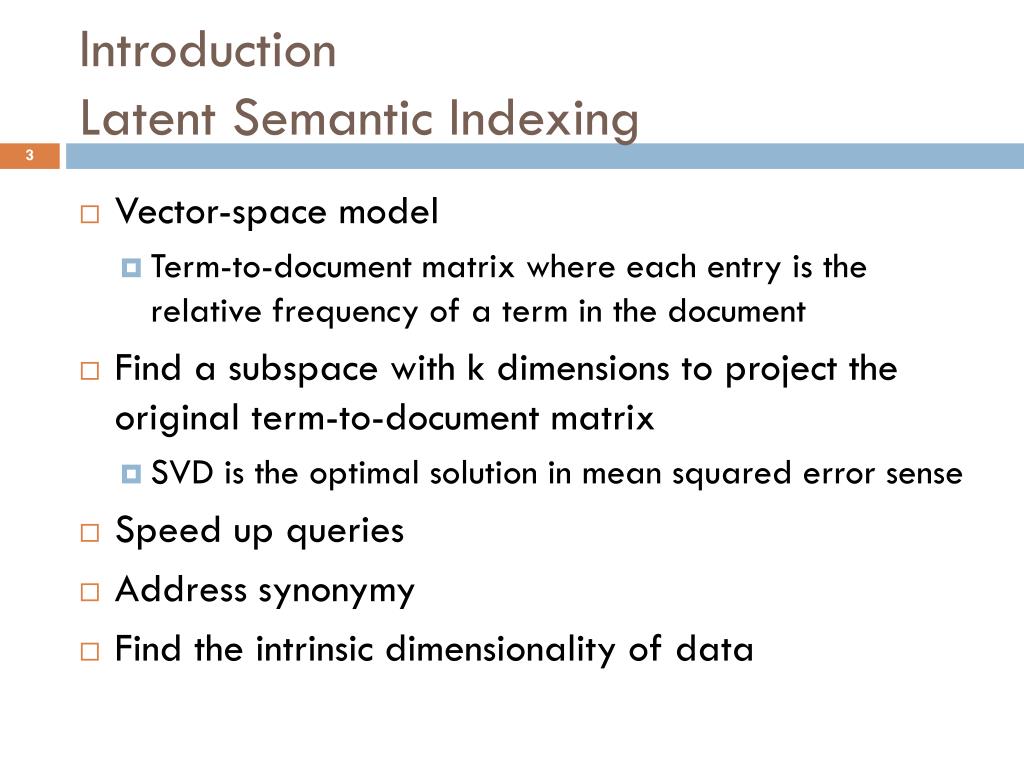
Ppt Dimensionality Reduction By Random Projection And Latent Semantic Lsa (latent semantic analysis) also known as lsi (latent semantic index) lsa uses bag of word (bow) model, which results in a term document matrix (occurrence of terms in a document). rows represent terms and columns represent documents. lsa learns latent topics by performing a matrix decomposition on the document term matrix using singular. There are many different mappings from high dimensional to low dimensional spaces. latent semantic indexing chooses the mapping that is optimal in the sense that it minimizes the distance ∆ . this setup has the consequence that the dimensions of the reduced space correspond to the axes of greatest variation.1. Latent semantic indexing (lsi) — natural language processing lecture. 6.1. latent semantic indexing (lsi) lsi has been developed in [ddf 90] as a method for topic extraction. for the description of lsi, assume that we have 5 simple documents, which contain the follwing words: document d1: cosmonaut, moon, car. document d2: astronaut, moon. Latent semantic indexing 2 summary: singular value decomposition (svd) m number of terms n number of documents c the term×document matrix of weights (our old w ij) –of rank r ( r ≤ min(m,n) ) cct and ctc: symmetric, share the same eigenvalues λ 1, λ 2,… –λ 1, λ 2, … are indexed in decreasing order svd: c = uΣvt.
Comments are closed.