Latent Semantic Indexing Explained With Examples Georgia Tech Cse6242

Latent Semantic Indexing Explained With Examples Georgia Tech This is a lecture video of the data and visual analytics (cse6242 cx4242) course at georgia tech. course website and lecture slides: poloclub.github . Georgia tech, college of computing. this course will introduce you to broad classes of techniques and tools for analyzing and visualizing data at scale. it emphasizes on how to complement computation and visualization to perform effective analysis. we will cover methods from each side, and hybrid ones that combine the best of both worlds.
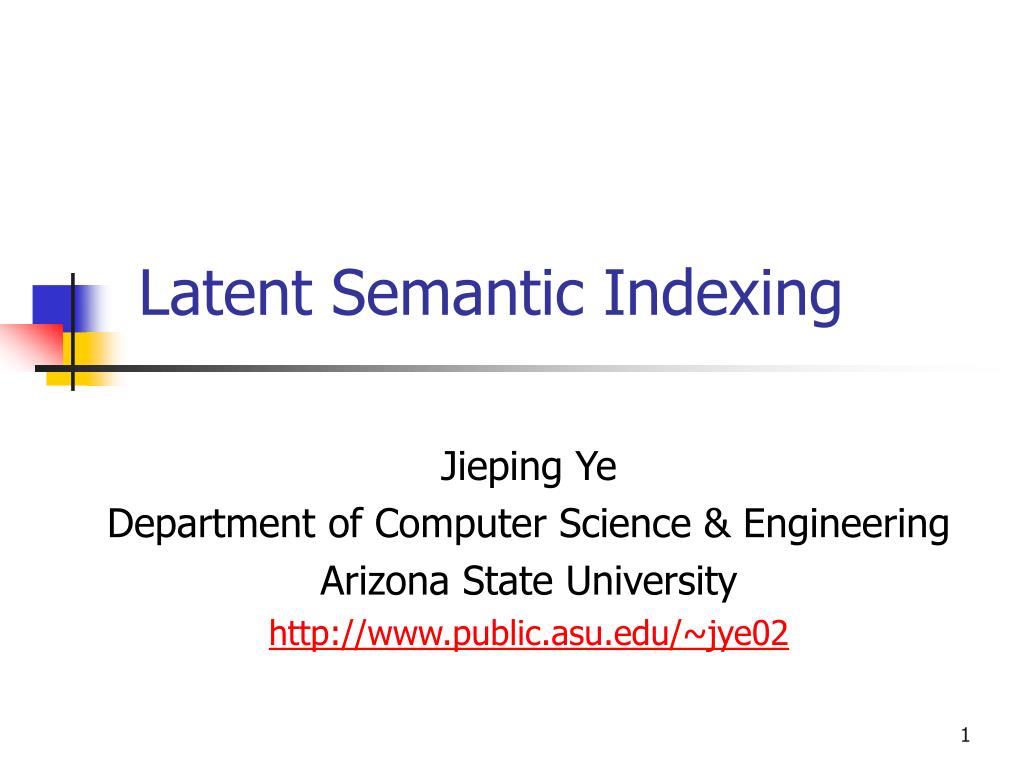
Ppt Latent Semantic Indexing Powerpoint Presentation Free Download Have taken cse 6040 and did very well in it (for georgia tech analytics students) have taken cs 1301 (for georgia tech analytics students) are proficient in at least one high level programming language (e.g., python, c , java) and are efficient with debugging principles and practices; if you are not, you should instead first take cse 6040 (for. Latent semantic indexing (lsi) applies singular value decomposition (svd) for calculating the low dimensional topic space [ ms00]. svd calculates a factorisation of the term by document matrix a: (6.1) at×d = tt×nsn×n(dd×n)t, where t and d are the number of words and the number of documents, respectively and n = min(t, d). Latent semantic indexing (lsi) or latent semantic analysis (lsa) is a technique for extracting topics from given text documents. it discovers the relationship between terms and documents. lsi concept is utilized in grouping documents, information retrieval, and recommendation engines. lsi discovers latent topics using singular value decomposition. As its name implies, the latent semantic indexing can reveal latent (hidden) relationship between terms, documents, topic terms, and document topics. references edfors, o. (2010, april 6).
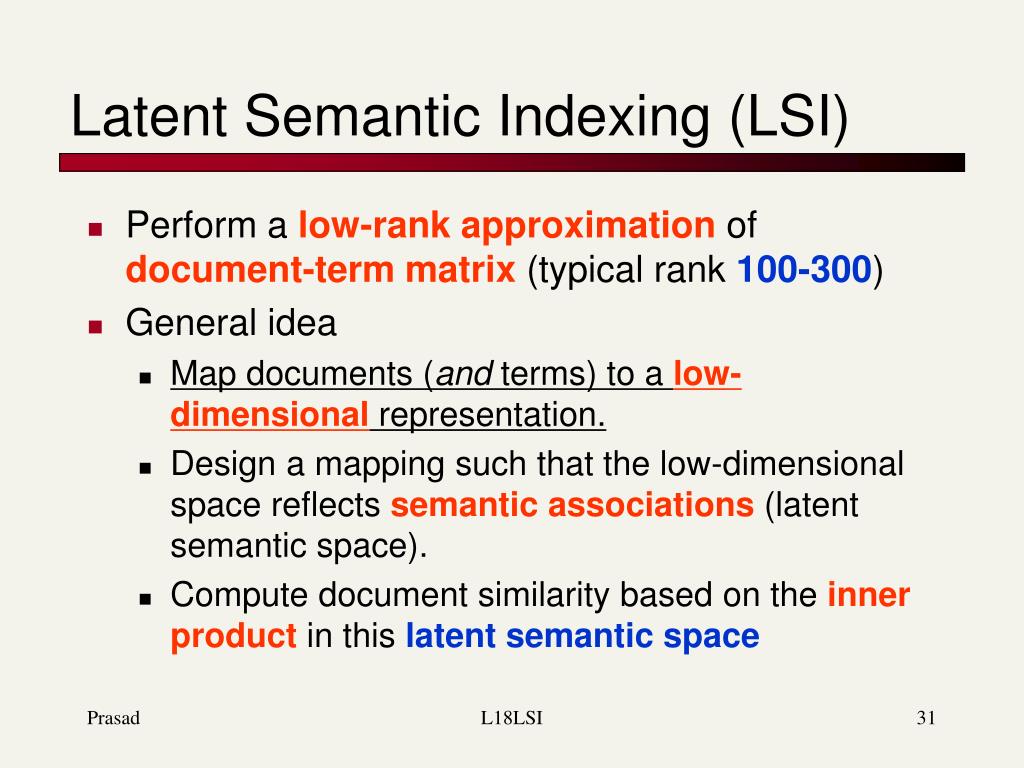
Ppt Latent Semantic Indexing Powerpoint Presentation Free Download Latent semantic indexing (lsi) or latent semantic analysis (lsa) is a technique for extracting topics from given text documents. it discovers the relationship between terms and documents. lsi concept is utilized in grouping documents, information retrieval, and recommendation engines. lsi discovers latent topics using singular value decomposition. As its name implies, the latent semantic indexing can reveal latent (hidden) relationship between terms, documents, topic terms, and document topics. references edfors, o. (2010, april 6). In the latent semantic space, a query and a document can have high cosine similarity even if they do not share any terms as long as their terms are semantically similar in a sense to be described later. we can look at lsi as a similarity metric that is an alternative to word overlap measures like tf.idf. the latent semantic space that we. The particular “latent semantic indexing” (lsi) analysis. queries are represented as pseudo document vectors that wehave tried uses singular value decomposition. we formed from weighted combinations of terms, and take a large matrix ofterm document association data and.
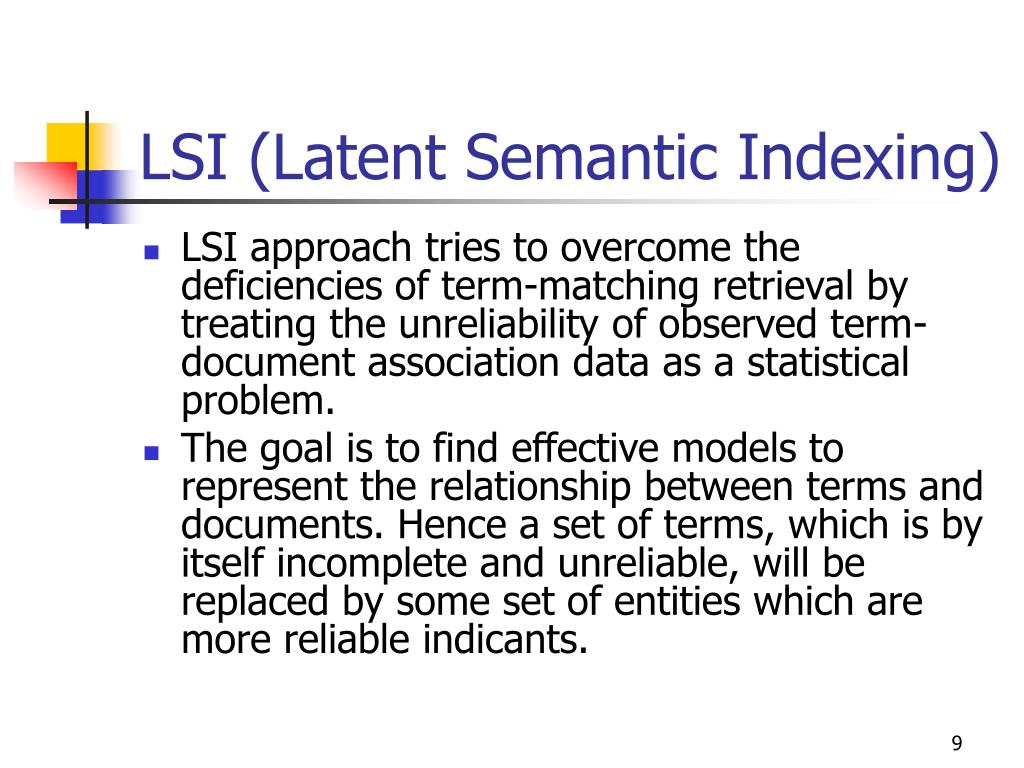
Ppt Latent Semantic Indexing Powerpoint Presentation Free Download In the latent semantic space, a query and a document can have high cosine similarity even if they do not share any terms as long as their terms are semantically similar in a sense to be described later. we can look at lsi as a similarity metric that is an alternative to word overlap measures like tf.idf. the latent semantic space that we. The particular “latent semantic indexing” (lsi) analysis. queries are represented as pseudo document vectors that wehave tried uses singular value decomposition. we formed from weighted combinations of terms, and take a large matrix ofterm document association data and.
Comments are closed.